Description
Smart modelling reshapes city planning; it can foresee multiple scenarios, provide customised solutions and improve decision-making through the application of data. This section introduces the use of smart modelling in the urban context. It focuses on three aspects: 1) possible data sources, 2) tools for data processing and 3) applications of mapping and modelling. It also covers other aspects, including third-party participation and technical expertise for smart modelling.
A good amount of data is essential for smart modelling. It is worth noting that building a smart model does not always entail the collection of new data. In fact, historical maps have proved very useful in the creation of flood-prevention models. In Da Nang, Vietnam, the city government, as a first step, collected historical flood maps, as well as past data on sea-level rise and climate change. It then developed a set of hydraulic parameters to build a predictive model.[i] Using the model, the city simulated changes in flooding patterns in certain areas and amended its urban development plan to mitigate flooding risks.
The adoption of third-party data also advances smart-modelling development. For instance, institutions including the US National Aeronautics and Space Administration (NASA) and the European Space Agency (ESA) provide satellite imagery with resolutions sufficient for land-cover mapping.[ii] Combined commercial and public data sources help solve complicated land-use issues[iii] (for example, by identifying hotspots of severe soil erosion and comparing historical and current land pictures).
Once the required data are in hand, the next step is data processing. In addition to big data analysis, machine learning[iv] and artificial intelligence (AI) are increasingly being used to analyse the urban context in real time. These technologies simplify the processing of datasets made up of cross-correlated information, and can thus observe urban development patterns dynamically.[v] First, city planners teach computers what to search for in different sources of data, then use machine-learning algorithms to integrate the results with administrative data to identify, for instance, flood-prone areas or illicit land use.
In Ho Chi Ming City, Vietnam, the government adopted rudimentary AI tools to mine satellite data sources, then used machine-learning algorithms that taught computers to automatically detect and classify different land cover types and land use. The results were overlaid with the city’s zoning data to create a map tracking potential zoning code violations.[vi]
Another commonly used data-processing tool is Geographic Information System (GIS) technology. Using GIS, planners can analyse and visualise real-world geographical data on digital maps and integrate them with different geographic components based on their needs.[vii] GIS technologies, along with 3D simulation, are being used for building-height control, climate-change adaption and development planning.[viii] This is usually known as “digital twinning” – creating a virtual replica of the actual environment, including physical objects, behaviours and processes. This technology helps cities, or even individual utilities, enhance data capture and real-time visualisation.
The Swedish city of Uppsala, for example, wanted to preserve its natural habitats while addressing a housing shortage. [ix] To increase the sustainability of its development plan, the city government adopted an interactive 3D model built on a digital elevation map captured by Lidar scanning.[x] The model helped stakeholders visualise how traditional infrastructure could be built in harmony with nature.
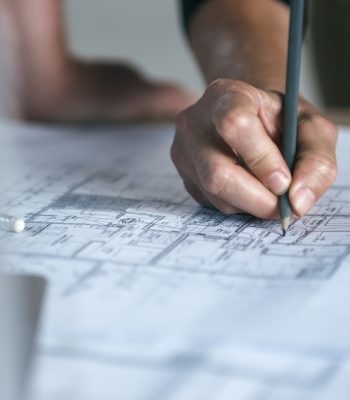
Resource implications and key requirements
Sufficient data
Smart models analyse past patterns to simulate future patterns, and the amount of data is a key element in a model’s capability. To develop a model that contains few data layers, cities may only need data from internal sources. If cities wish to address more complex issues, however, such as housing or urban planning, using data from various sources becomes key to success. In Honolulu, Hawaii, the government sought to tackle illegal apartment rentals by redesigning city zoning. It collected citywide Lidar datasets between 1990 and 2017 and integrated them with imagery, 3D elevations and GIS data.[xi] Multiple 3D data services were created to enable the visualisation of the urban context, so city staff could obtain more details on the urban development plan.
Technical expertise
Developing and maintaining smart models requires high-level technical expertise. In practice, cities with sufficient IT capacity will designate a unit to coordinate different agencies and oversee the project. For instance, municipal departments may need to transform administrative and regulatory data into a set of parameters to successfully launch a planning model.
In addition to internal resources, technical support from external experts is often needed to develop and maintain modelling products. The city of Da Nang, for example, set up committees on different levels to collaborate with a non-governmental organisation. The city obtained technical assistance and funds to support its hydraulic model, which cost about US$ 367,000.
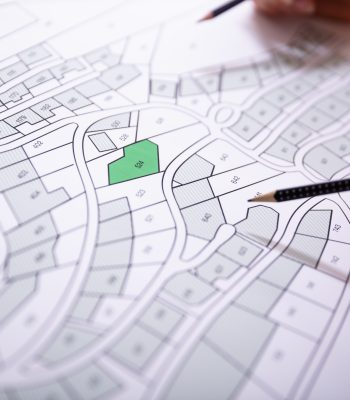
Potential private-sector participation
Smart models provide cities with an intuitive tool for evaluating, planning and improving cities’ development plans. Not only can planners simulate urban scenarios and test alternatives in a virtual setup, they can choose to communicate urban planning information with stakeholders through the visualisation of data. By doing so, planners and researchers have more opportunities to cooperate and add value to cities’ smart model development.
In Boston, several agencies worked together to transform the city’s administrative data into a set of parameters, the foundation of its 3D smart model. Using the model, cross-departmental teams could establish shared projects and set common indicators. The 3D smart model was open to city planners and citizens, allowing private investors to locate suitable areas easily and to invest in real estate or commercial infrastructure.[xii] In addition, based on the 3D smart model, the Boston planning and development agency and the Massachusetts Institute of Technology (MIT) successfully created an urban energy model that would facilitate energy savings and the roll-out of microgrids.[xiii]
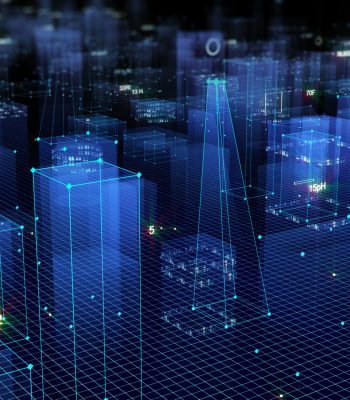
Obstacles to implementation and potential solutions
Cities wanting to adopt smart models may suffer from a lack of relevant expertise, including but not limited to technical skills. City agencies may lack the IT talent to initiate a model’s development, not to mention provide technical support for updates. To meet this challenge, cities can work with private companies and leverage their expertise. However, cities should also grasp the importance of enhancing in-house operational skills. Technical professionals play a crucial role in the formulation of a smart city strategy, as well as in the operation and maintenance of technical tools. Therefore, city governments ought to set a budget that allows departments to train their staff and recruit extra professionals to develop strategies, familiarise themselves with new technologies and maintain them. [xiv]
Alternatively, the lack of technical expertise may translate into a reluctance to use smart models. This may well occur among city staff who have to shoulder the extra workload. Not only do they need to learn to use the new tool, but they are also at times responsible for supporting its maintenance (for example, integrating administrative data into the model). Introducing performance-based evaluation and educational programmes may help staff adjust to the new planning system.
Another barrier that cities need to overcome is conflicts of interest. It is crucial to understand that while smart models allow city governments to make insightful changes and/or enhance planning, other stakeholders may perceive the opposite to be true. One such scenario could involve the government requiring residents living in flood-prone areas to refurbish their properties or migrate to safer zones, but residents refusing to comply. The city of Da Nang avoided such conflict by providing residents with proper financial and technical support for refurbishment, which allowed them to survive a catastrophic typhoon unscathed.[xv] Some cities chose to share their smart models to gain the public’s trust. Both Boston, Massachusetts and Ho Chi Min City, Vietnam opened up their land-use information to the public and, in return, the public informed the government about potential breaches of the law. This virtuous circle would help eliminate illicit land use in the long run and unleash cities’ growth potential on various fronts.
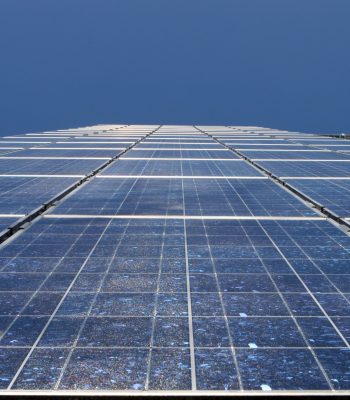
References
[i] S. Tyler, V.G.P. Tran, T.K.H. Nguyen, V.T. Huynh, V.D. Tran, P.T. Nghiem et al. (2016), Urban Development and Flood Risk in Vietnam: Experience in Three Cities, Boulder, CO: ISET-International. Available at: https://2eac3a3b-5e23-43c7-b33c-f17ad8fd3011.filesusr.com/ugd/558f8a_98db521f15aa4d7096e1b9be83655df8.pdf.
[ii] R. Goldblatt, K. Kaiser, H.T.L. Tran and K. Vu (2018), “Artificial intelligence for smart cities: insights from Ho Chi Minh City’s spatial development”, Washington, DC: World Bank. Available at: https://blogs.worldbank.org/opendata/artificial-intelligence-smart-cities-insights-ho-chi-minh-city-s-spatial-development.
[iii] Ibid.
[iv] “Machine learning is an application of artificial intelligence (AI) that provides systems the ability to automatically learn and improve from experience without being explicitly programmed. Machine learning focuses on the development of computer programs that can access data and use it to learn for themselves.” Expert.ai (2020), “What is Machine Learning? A Definition”. Available at: https://www.expert.ai/blog/machine-learning-definition/.
[v] G. Giannakodakis (2018), “Urban planning & property development using artificial intelligence”, Adelaide, Australia: PointData. Available at: https://pointdata.com.au/urban-planning-property-development-using-ai/.
[vi] Ibid.
[vii] Esri (n.d.), “What is GIS?” Aylesbury, UK. Available at: https://www.esri.com/en-us/what-is-gis/overview.
[viii] Singapore Land Authority (2015), “Leveraging 3D Mapping for Planning and Land Management”, Singapore. Available at: https://unstats.un.org/unsd/geoinfo/rcc/docs/rccap20/28_Presentation_Singapore_Kheng%20Peng%20Soh.pdf.
[ix] B. Patrick (2021) “Uppsala Creates a Detailed Digital Twin to Enhance Sustainability”, Aylesbury, UK: Esri. Available at: https://www.esri.com/about/newsroom/blog/uppsala-sustainable-development/.
[x] Ibid.
[xi] B. Patrick (2019) “Honolulu Planners Visualize Housing Patterns with an Eye on Affordability”, Aylesbury, UK: Esri. Available at: https://www.esri.com/about/newsroom/blog/honolulu-planners-visualize-urban-development-patterns/.
[xii] Boston Planning and Development Agency (2018), “BPDA Launches a Web-Based 3D Smart Model for Public Use”, Boston, MA. Available at: http://www.bostonplans.org/news-calendar/news-updates/2018/5/8/bpda-launches-a-web-based-3d-smart-model-for-publi.
[xiii] Boston Planning and Development Agency (n.d.), “Boston Community Energy Study”, Boston, MA. Available at: https://boston.maps.arcgis.com/apps/MapSeries/index.html?appid=33c30cb10c294edabf30da51141893cf.
[xiv] Roweb (2021), “Roweb launches SmartCity: the complete solution for safer and simplified public services.” Pitesti, Romania. Available at: https://www.roweb.ro/blog/roweb-launches-smartcity-complete-solution-safer-simplified-public-services/.
[xv] ACCCRN City Project (2013), Asian Cities Climate Change Resilience Network, Bangkok: Rockefeller Foundation. Available at: https://www.rockefellerfoundation.org/wp-content/uploads/8ff925b8-2254-4b71-a7fb-6082464b844e-acccrn-cities.pdf.